Deep learning has revolutionized the way computers learn and make decisions. Deep learning is the technology behind smart assistants, self-driving cars, and many other cool innovations we see today. As this field grows, new and exciting trends are emerging.
The emerging trends in deep learning research include transformer models, self-supervised learning, federated learning, explainable AI, energy-efficient deep learning, and edge computing applications.
In this blog, we’ll explore six key emerging trends in deep learning research. Whether you’re new to AI or just curious about what’s next, this guide will help you understand cutting-edge developments in simple terms.
Table of Contents
- 1. Transformer Models
- 2. Self-Supervised Learning
- 3. Federated Learning
- 4. Explainable AI
- 5. Energy-Efficient Deep Learning
- 6. Deep Learning in Edge Computing
- FAQ’s
- Conclusion
1. Transformer Models
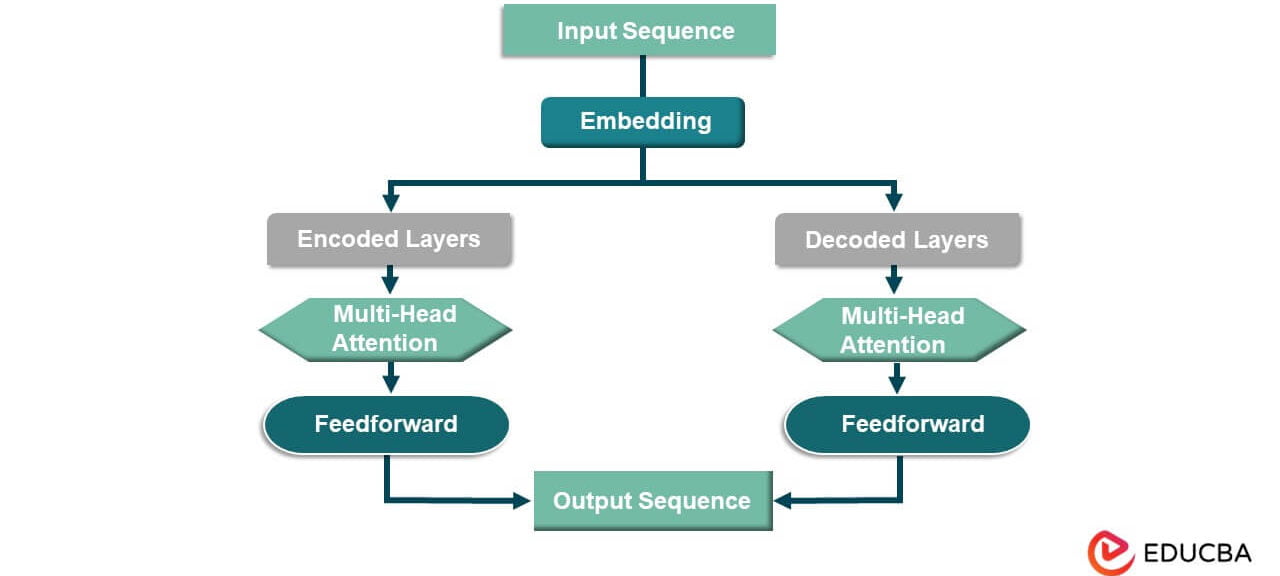
Transformer models are like super-smart language processors. They’re changing the game in deep learning, especially for tasks involving language.
Here’s what makes them special:
- They can understand context really well
- They’re great at handling long sequences of data
- They’ve led to breakthroughs in language translation and text generation
Think of transformers as expert readers who can grasp the meaning of a whole book, not just individual sentences.
They’re behind popular AI tools like GPT-3, which can write human-like text. Researchers are now applying transformers to other areas like image and video processing, opening up exciting new possibilities.
2. Self-Supervised Learning
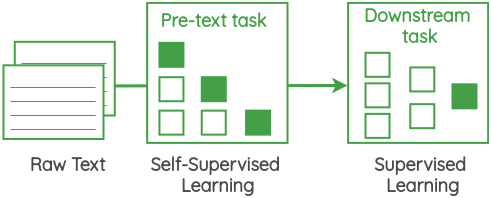
Self-supervised learning is a clever way to teach AI without tons of labeled data.
It works like this:
- The AI looks at a bunch of unlabeled data
- It figures out patterns and relationships on its own
- It uses these insights to learn and improve
This method is super helpful because labeling data can be time-consuming and expensive. With self-supervised learning, AI can learn from much larger datasets.
It’s being used to improve things like:
- Image recognition
- Speech understanding
- Robotic control
This approach is helping AI become more flexible and adaptable, just like humans!
3. Federated Learning
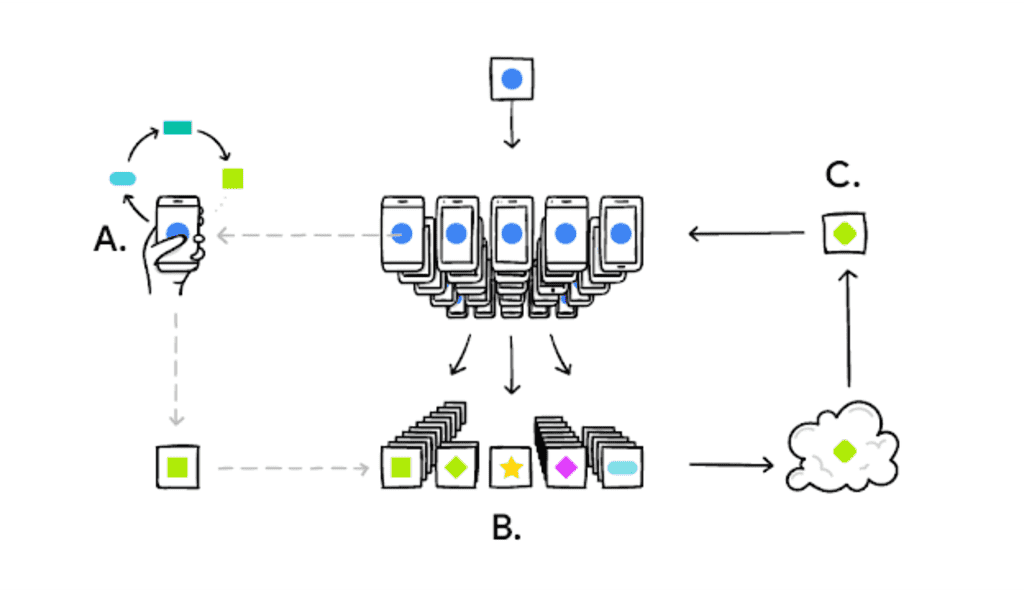
Federated learning is all about privacy. It lets AI learn from data without actually seeing it.
Here’s how it works:
- The AI model is sent to where the data is (like your phone)
- It learns from the local data
- Only the learning results are sent back, not the raw data
This is great because:
- Your personal info stays on your device
- The AI still gets smarter
- It works even with poor internet connections
Federated learning is perfect for apps that deal with sensitive info, like health data or financial records. It’s a win-win: better AI and better privacy!
4. Explainable AI
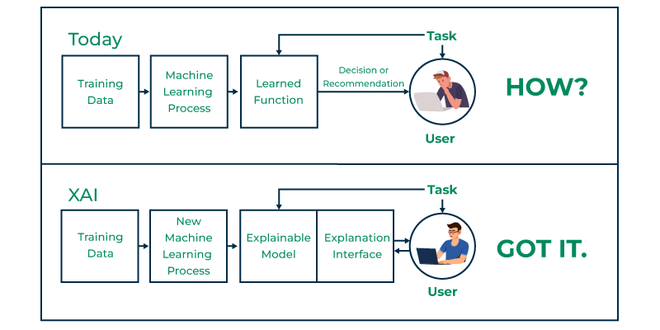
Explainable AI is about making deep learning models that we can understand. Right now, many AI systems are “black boxes” – we don’t know how they make decisions. Explainable AI aims to change that.
Why is this important?
- It builds trust in AI systems
- It helps us spot and fix biases
- It’s crucial to use AI in fields like healthcare and finance
Researchers are working on tools to:
Visualize what AI models are focusing on Provide clear reasons for AI decisions Create simpler models that are easier to interpret
The goal is to make AI that’s not just smart, but also transparent and trustworthy.
5. Energy-Efficient Deep Learning
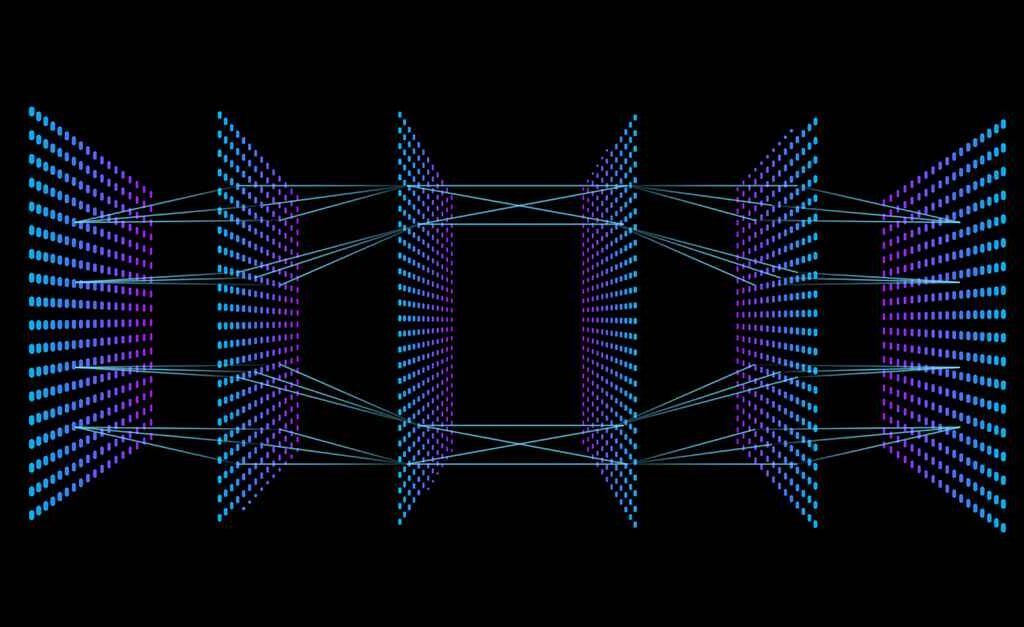
Deep learning can be a power hog. That’s why researchers are working on making it more energy-efficient.
Here’s what they’re doing:
- Creating smaller, faster models
- Developing new hardware that uses less power
- Finding ways to train AI with fewer computations
This trend is important because:
- It reduces the environmental impact of AI
- It makes AI more accessible on everyday devices
- It cuts down on the costs of running AI systems
By making deep learning greener, we can use AI in more places without worrying about high energy bills or environmental damage.
6. Deep Learning in Edge Computing
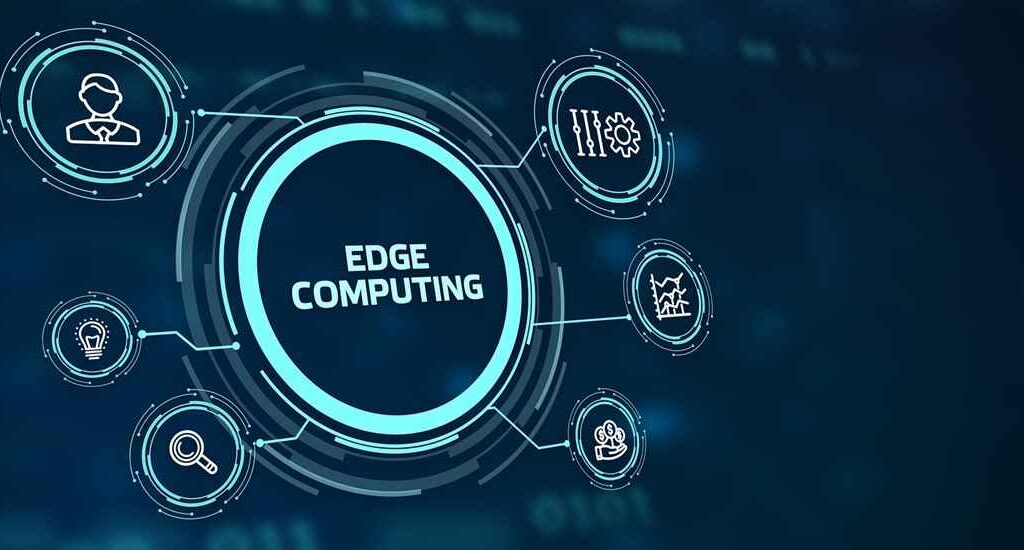
Edge computing brings AI closer to you. Instead of sending data to far-away servers, edge computing processes data right where it’s collected.
This is big for deep learning because:
- It reduces delays in AI responses
- It works even without an internet connection
- It helps keep your data more private
Examples of edge AI:
- Smart home devices that understand voice commands
- Self-driving cars that make quick decisions
- Phones that can do face recognition without the cloud
This trend is making AI faster, more reliable, and more private in our everyday lives. This trend is not only seen in smart home devices and self-driving cars but also in various audio processing tools, which use deep learning models to operate efficiently on edge devices.
FAQ’s
The latest developments in deep learning include transformer models, self-supervised learning, federated learning, explainable AI, energy-efficient models, and edge computing applications.
Deep learning is trending because it can solve complex problems in areas like language processing, image recognition, and decision-making.
Interdisciplinary projects, Real-world problem-solving, Technology integration, Collaborative online projects, and Personalized learning paths these are 5 emerging trends in project-based learning.
Conclusion
In conclusion, the emerging trends in deep learning research are shaping the future of AI. From smarter language models to privacy-focused learning, these advances are making AI more powerful and useful. As researchers continue to push boundaries, we can expect even more exciting developments. Whether you’re a tech enthusiast or just curious, keeping an eye on these trends will give you a glimpse into the incredible potential of deep learning.