AI is incredibly smart, but it’s not perfect. There is uncertainty in AI that we need to understand. AI systems can make mistakes or give unreliable outputs sometimes.
Uncertainty in AI comes from things like inaccurate data, simplified models, and unpredictable environments. While AI is powerful, its uncertainty factor means it has limitations.
In this blog post, we’ll explore what is uncertainty in AI, the different types of uncertainty in AI systems, its examples, and how it impacts AI’s capabilities and trustworthiness. Let’s get started!
Table of Contents
- Understanding Uncertainty in AI
- Types of Uncertainty in AI
- Examples of Uncertainty in AI
- How Uncertainty Impacts AI’s Capabilities and Trustworthiness
- Reasoning Under Uncertainty in AI
- Quantifying Uncertainty in AI
- Causes of Uncertainty in AI
- Techniques for Handling Uncertainty in AI
- Conclusion
Understanding Uncertainty in AI
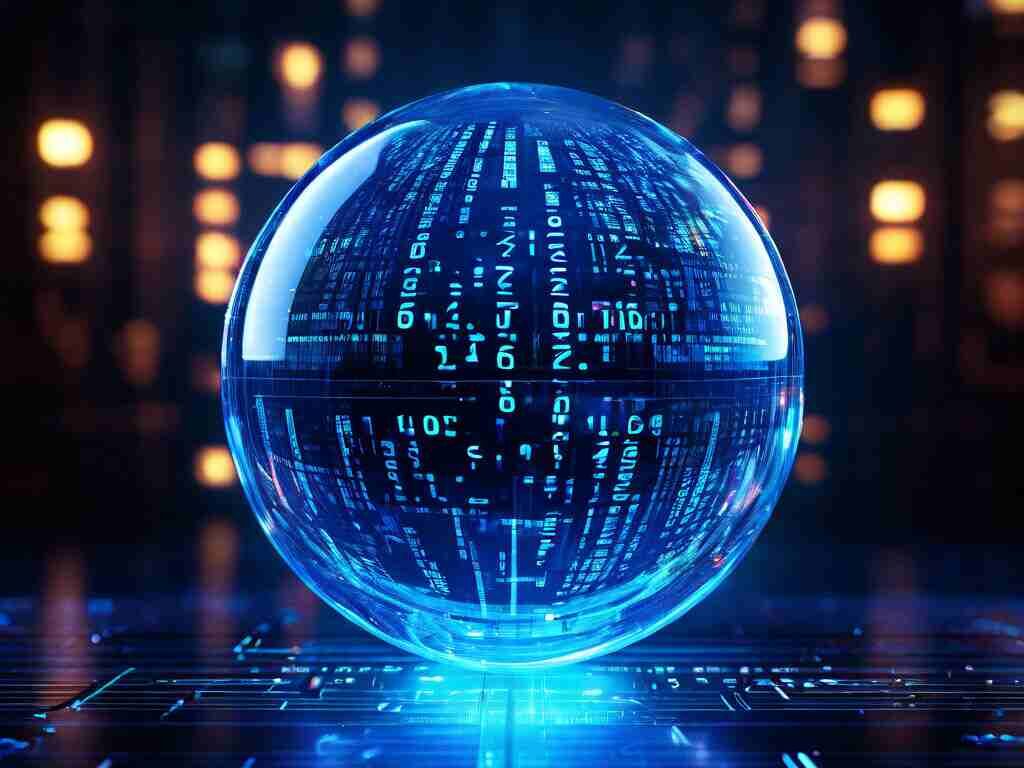
Uncertainty in AI refers to the doubt or skepticism around an AI system’s predictions or outputs. Even advanced AI is not 100% accurate or reliable all the time. There is always some uncertainty involved.
This uncertainty can come from various sources like data quality issues, overly simplified models, or dynamic real-world conditions. Unlike calculators which give perfectly precise results, AI models have to deal with ambiguity, noise, and approximations.
As a result, there is an inherent uncertainty factor in AI systems. This uncertainty manifests as incorrect outputs, unintuitive results, or a lack of confidence in the AI’s capabilities in certain situations.
Types of Uncertainty in AI
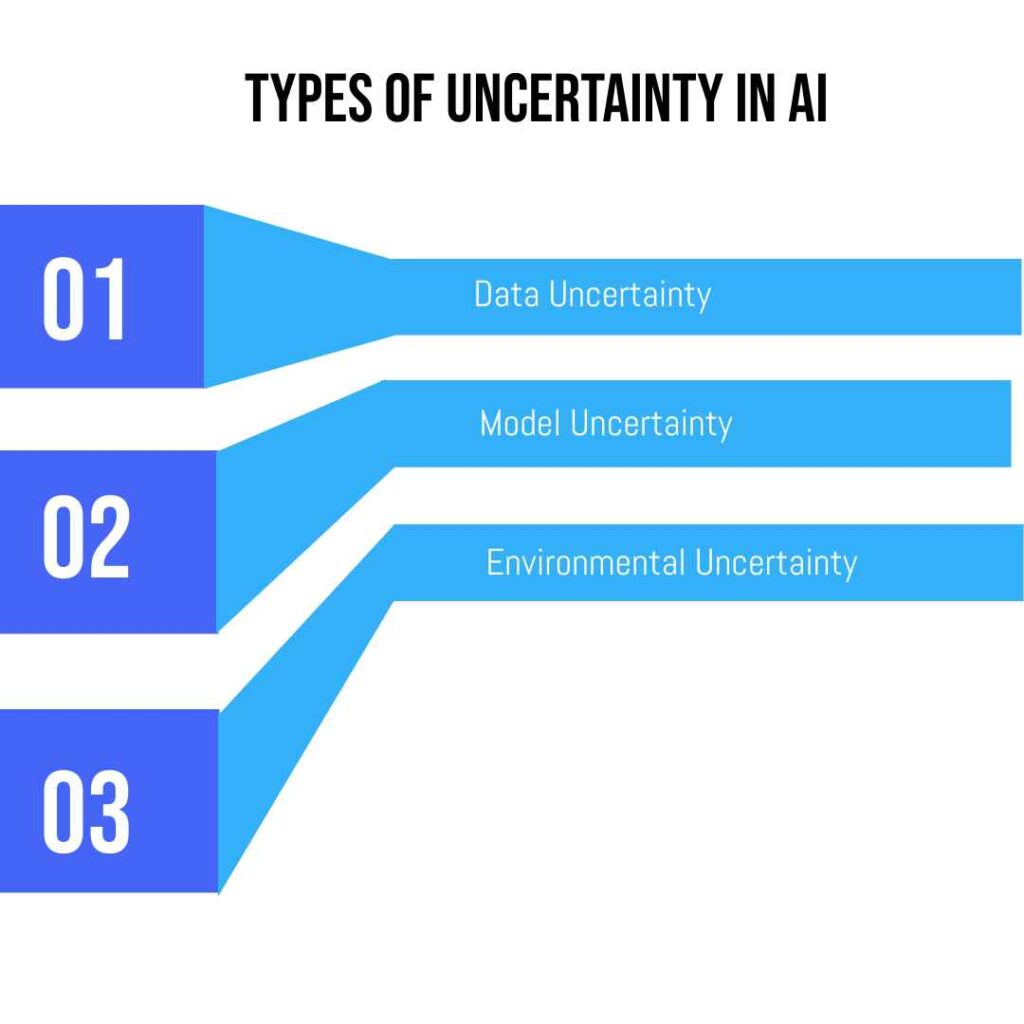
There are several key types of uncertainty that AI systems can face:
Type 1: Data Uncertainty
The data used to train an AI model can be incomplete, noisy, or biased. If the training data is low-quality or lacking in diversity, it introduces uncertainty into the AI’s predictions. Inaccurate or underrepresentative data leads to unreliable outputs.
Type 2: Model Uncertainty
All AI models involve approximations and assumptions to simplify the problem. These approximations create uncertainty, as the model may not perfectly represent the real-world scenario. More complex models reduce this uncertainty but can’t eliminate it completely.
Type 3: Environmental Uncertainty
Most AI models are designed for specific situations. But the real world is messy and open-ended. Unexpected changes or new contexts that deviate from the AI’s training introduce uncertainty in its behavior and outputs.
By understanding these types of uncertainties, developers can try to detect and mitigate their impacts through better data practices, more sophisticated modeling techniques, and continual learning systems.
Examples of Uncertainty in AI
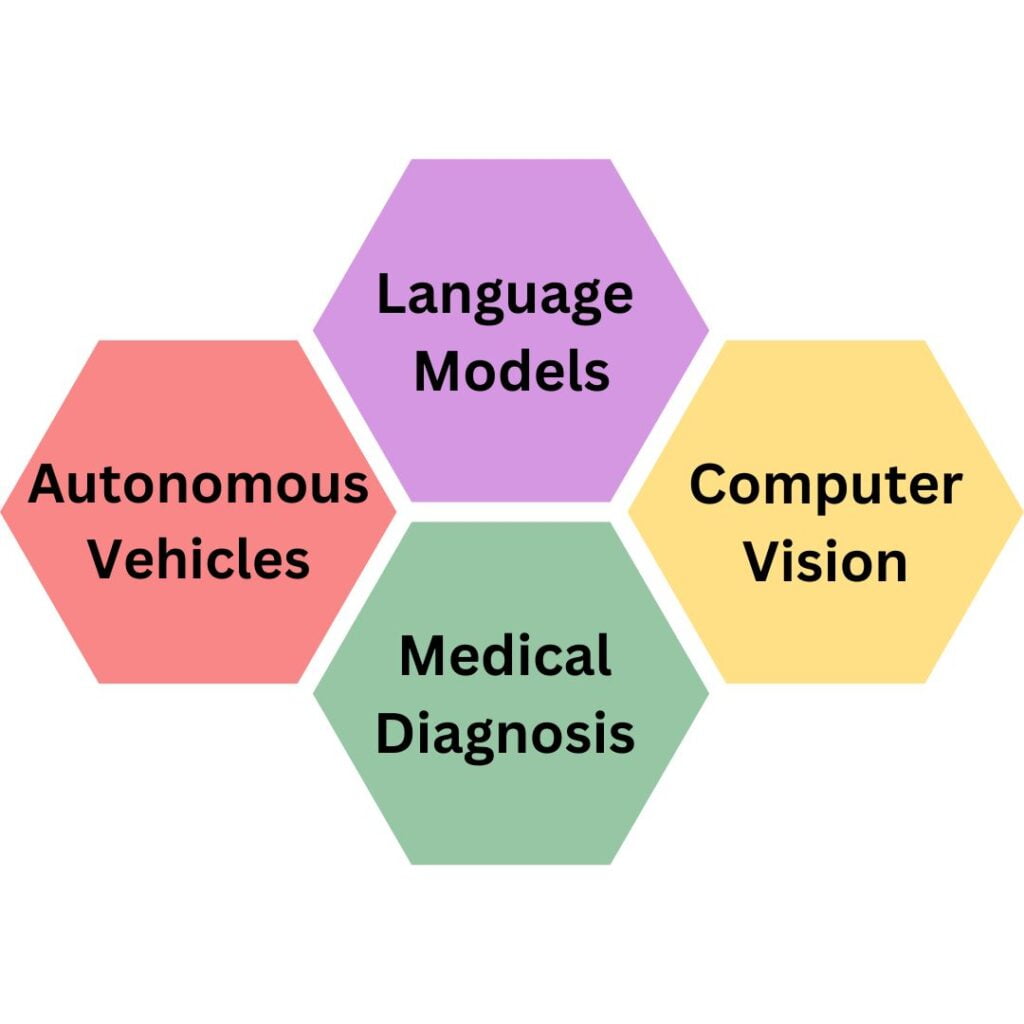
Uncertainty in AI can manifest in many different ways across various applications. Here are some common examples:
Example 1: Language Models
AI language models like chatbots or translation tools can produce uncertain outputs when faced with ambiguous text, idioms, or lack of context. This uncertainty leads to nonsensical or inaccurate responses.
Example 2: Computer Vision
While AI vision is highly advanced, it struggles with uncertainty caused by visual noise, occlusions, or overlapping objects. This can result in missed or incorrect object detections.
Example 3: Medical Diagnosis
AI systems can diagnose diseases from medical scans with high accuracy. However, there is still uncertainty when dealing with rare conditions, artifacts, or ambiguous data inputs leading to potential misdiagnoses.
Example 4: Autonomous Vehicles
Self-driving cars use AI to perceive and navigate environments. However, their perception is uncertain in harsh weather, construction zones, or with broken traffic signals, increasing accident risks.
These examples highlight how uncertainty in AI, stemming from complex data, approximations, or evolving situations, can significantly impact the reliability and safety of these systems in the real world.
How Uncertainty Impacts AI’s Capabilities and Trustworthiness
Uncertainty in AI systems can have major impacts on their capabilities and how much we can trust their outputs. Some key ways uncertainty affects AI include:
Reliability Issues
When there is high uncertainty, AI systems become less reliable and consistent. Their predictions and decisions may frequently be incorrect or contradict previous outputs on similar inputs.
Safety Risks
For AI applied in high-stakes domains like self-driving cars or medical diagnosis, uncertainty increases the risks of failures that could jeopardize safety. Uncertain AI is not trustworthy enough for life-or-death scenarios.
Unintuitive Behavior
Uncertainty can cause AI models to exhibit surprising, counter-intuitive behaviors that confuse and undermine user trust. Their responses may seem random or illogical.
Decision Bottlenecks
AI uncertainty reduces our ability to fully automate decision processes. Humans still need to audit uncertain AI outputs before acting, reducing efficiency gains.
Fundamentally, high levels of uncertainty impose hard limits on AI’s capabilities in the real world. An uncertainty factor means the AI isn’t skilled or knowledgeable enough to handle complex, stakes situations reliably without human oversight. Managing this uncertainty is essential for more trusted and capable AI.
Reasoning Under Uncertainty in AI
Even with uncertainty in AI systems, they still need to operate and make decisions. AI models use probabilistic reasoning techniques like Bayesian networks to make predictions and choices under uncertainty.
Instead of definite outputs, they provide probability distributions over possible outcomes. This allows capturing the uncertainty while still being able to reason and function.
Quantifying Uncertainty in AI
It’s critical to quantify the uncertainty in an AI model’s predictions. Metrics like predictive variance, confidence intervals, and entropy are used to measure the degree of uncertainty.
Higher uncertainty is flagged for human review. Quantifying uncertainty helps understand an AI’s limitations and decide when to trust its outputs.
Causes of Uncertainty in AI
There are several root causes of uncertainty in AI models. Limited training data, oversimplified assumptions, discrimination issues, and inability to transfer learning can all introduce uncertainties. Evolving real-world conditions and lack of experience in certain areas also increase uncertainty for AI systems.
Techniques for Handling Uncertainty in AI
AI developers use different techniques to mitigate and manage uncertainty like:
- Ensemble methods that combine multiple models
- Online/continual learning to adapt to new data
- Explicit modeling of uncertainties
- Hybrid human-AI decision workflows
- Abundant training data from diverse sources While uncertainty cannot be eliminated completely, these methods allow the development of more robust and reliable AI systems.
These are the techniques used for handling uncertainty in AI.
Conclusion
In conclusion, uncertainty is an inherent aspect of modern AI systems that must be recognized and addressed. From data quality issues to evolving real-world conditions, there are various sources that introduce uncertainties in AI models.
Understanding and quantifying this uncertainty factor is crucial for identifying an AI’s limitations and deciding when human oversight is required. As AI capabilities grow, developing robust techniques to reason under uncertainty will be vital for developing trustworthy and reliable artificial intelligence.

Ajay Rathod loves talking about artificial intelligence (AI). He thinks AI is super cool and wants everyone to understand it better. Ajay has been working with computers for a long time and knows a lot about AI. He wants to share his knowledge with you so you can learn too!