In today’s fast-paced world, AI is transforming how machines interact with data. Many technologies turn static models into more intelligent, dynamic tools to solve complex problems.
RAG AI technology turns a static model into a dynamic tool that searches for the most relevant information in real-time. This makes the model adaptable and flexible, unlike traditional static models.
In this blog, we’ll explain how RAG AI works, its benefits, and why it’s important for improving AI applications. Keep reading to learn more about this exciting technology!
Table of Contents
- What is a Static Model in AI?
- What Does It Mean to Turn a Static Model Into a Dynamic Tool?
- Introduction to RAG (Retrieval-Augmented Generation) AI Technology
- How Does RAG AI Technology Turn Static Models Into Dynamic Tools?
- Benefits of Using RAG AI Technology
- Other AI Technologies That Make Models Dynamic
- Conclusion
What is a Static Model in AI?
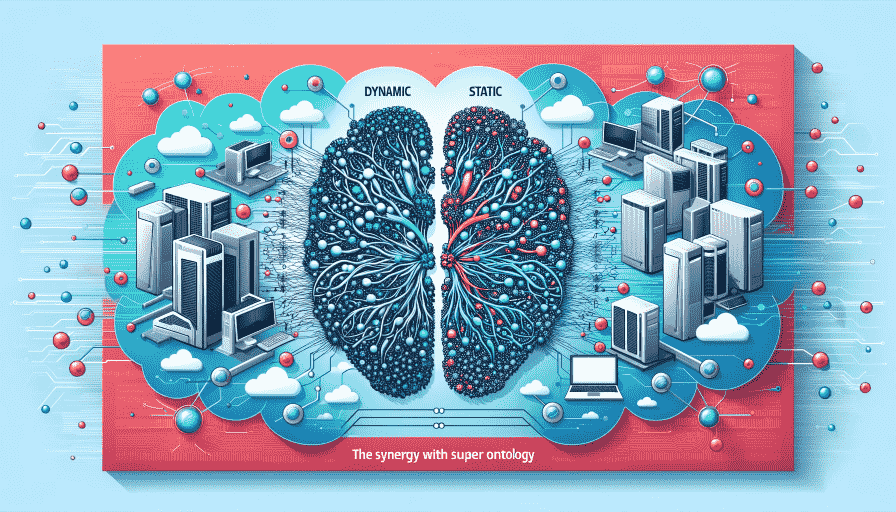
A static model in AI is a type of machine learning model that doesn’t change once it’s trained. It learns from a fixed set of data and remains the same over time.
This means the model cannot adapt to new data or update itself with new information. While it can make predictions based on what it has learned, it becomes less useful if the data changes.
For example, a static model trained on last year’s data may not give accurate results for this year’s trends, making it less effective in real-world applications.
What Does It Mean to Turn a Static Model Into a Dynamic Tool?
Turning a static model into a dynamic tool means making it flexible and able to adapt to new information. Unlike a static model, which stays the same after training, a dynamic tool can update itself with fresh data.
A dynamic tool continuously learns and improves its performance. It can respond to real-time changes and provide more accurate and relevant results.
This shift from static to dynamic is important because it allows AI systems to stay current, handle new situations, and be more useful in practical applications like chatbots, virtual assistants, or recommendation systems.
Introduction to RAG (Retrieval-Augmented Generation) AI Technology
RAG (Retrieval-Augmented Generation) AI technology combines two main parts: a static model and a retrieval system. The static model generates answers, while the retrieval system searches for up-to-date information from external sources.
This combination allows the AI to give more accurate and relevant responses. Instead of relying only on old data, RAG can pull new information whenever needed. This makes it more flexible and useful than a basic static model.
RAG is widely used in AI applications that require real-time information, like chatbots, virtual assistants, and search engines.
How Does RAG AI Technology Turn Static Models Into Dynamic Tools?
RAG technology transforms a static model into a dynamic tool by allowing it to search and retrieve fresh data. Here’s how it works:
First, the static model is trained on a fixed set of data. When a user asks a question, the RAG system searches external databases or knowledge sources for the most relevant information. It then combines this new data with the static model’s existing knowledge to generate an updated, accurate response.
This process makes the model more adaptable and able to respond to real-time changes, which is essential for modern AI applications.
Benefits of Using RAG AI Technology
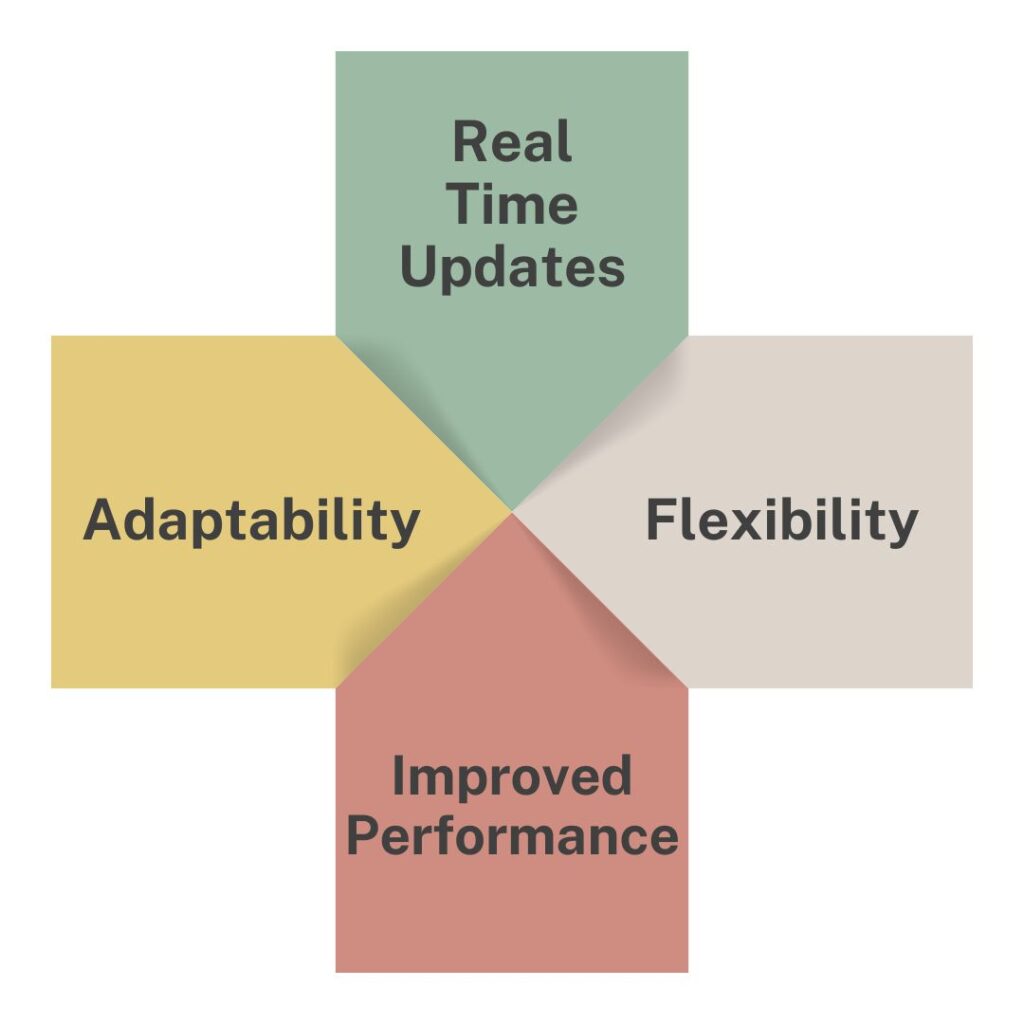
Here are the benefits of using RAG AI technology:
1. Real-time updates
RAG can fetch the latest information, making it much more accurate than static models.
2. Flexibility
Since RAG retrieves new data, it can handle a wide range of topics without needing constant retraining.
3. Improved performance
By combining old and new data, RAG provides better responses, improving user experience.
4. Adaptability
RAG can adjust to new situations, making it useful for dynamic environments like customer support or content generation.
Other AI Technologies That Make Models Dynamic
While RAG is one method to make AI models dynamic, there are other technologies as well:
Active Learning
This allows the model to request more data for training when needed, improving accuracy over time.
Reinforcement Learning
In this method, the model learns by trial and error, adjusting its behavior based on rewards or penalties.
Transfer Learning
This involves using a pre-trained model and fine-tuning it with new data to handle specific tasks.
These technologies also help make AI systems more flexible and adaptable to changing environments.
Conclusion
In summary, RAG AI technology turns static models into dynamic tools by combining old data with new, relevant information. It provides real-time updates, making AI more accurate and adaptable. Other technologies like Active Learning, Reinforcement Learning, and Transfer Learning also offer ways to create flexible AI models. Together, these advancements help improve the effectiveness and usability of AI systems in real-world applications.

Ajay Rathod loves talking about artificial intelligence (AI). He thinks AI is super cool and wants everyone to understand it better. Ajay has been working with computers for a long time and knows a lot about AI. He wants to share his knowledge with you so you can learn too!
Hi there to all, for the reason