Ontological Engineering in AI is a fascinating field that bridges knowledge representation and advanced technology. This innovative approach helps machines understand and organize complex information more effectively.
Ontological Engineering in Artificial Intelligence is the process of developing formal knowledge representations that enable intelligent systems to model and reason about specific domains with precision and clarity.
By breaking down intricate concepts into structured frameworks, this technique empowers AI systems to learn, interpret, and communicate knowledge in more meaningful and intelligent ways.
Table of Contents
- Understanding Ontological Engineering
- What is Ontological Engineering in AI?
- Why is Ontological Engineering Important in AI?
- Challenges in Ontological Engineering for AI
- Future of Ontological Engineering in AI
- Conclusion
Understanding Ontological Engineering
Ontological engineering is a systematic approach to creating structured representations of knowledge in a specific domain. It involves designing comprehensive frameworks that help machines understand and organize complex information with precision and clarity.
By mapping out relationships between concepts, ontological engineering enables artificial intelligence systems to process and interpret data more effectively, bridging the gap between human understanding and machine intelligence.
What is Ontological Engineering in AI?
Ontological Engineering in Artificial Intelligence is a specialized process of creating formal knowledge representations that enable AI systems to understand, organize, and reason about complex information within specific domains.
It involves developing structured frameworks that capture relationships between concepts, allowing machines to interpret data more intelligently.
These ontologies serve as blueprints that help AI systems learn, categorize, and make logical inferences about different types of information.
By mapping out intricate knowledge structures, ontological engineering empowers artificial intelligence to process and understand data with remarkable precision and depth.
Why is Ontological Engineering Important in AI?
Ontological engineering is crucial in advancing artificial intelligence by creating structured knowledge frameworks that enable machines to understand and process complex information more effectively.
By developing precise semantic models, AI systems can accurately categorize, relate, and reason about data. This approach helps overcome traditional limitations in machine learning and knowledge representation.
Key benefits include:
- Improved data interpretation and reasoning capabilities
- Enhanced machine learning and knowledge sharing
- More intelligent and context-aware AI systems
- Better decision-making through a comprehensive understanding
Ontological engineering bridges the gap between human knowledge and machine intelligence, allowing AI to transform raw data into meaningful, actionable insights across various domains.
Challenges in Ontological Engineering for AI
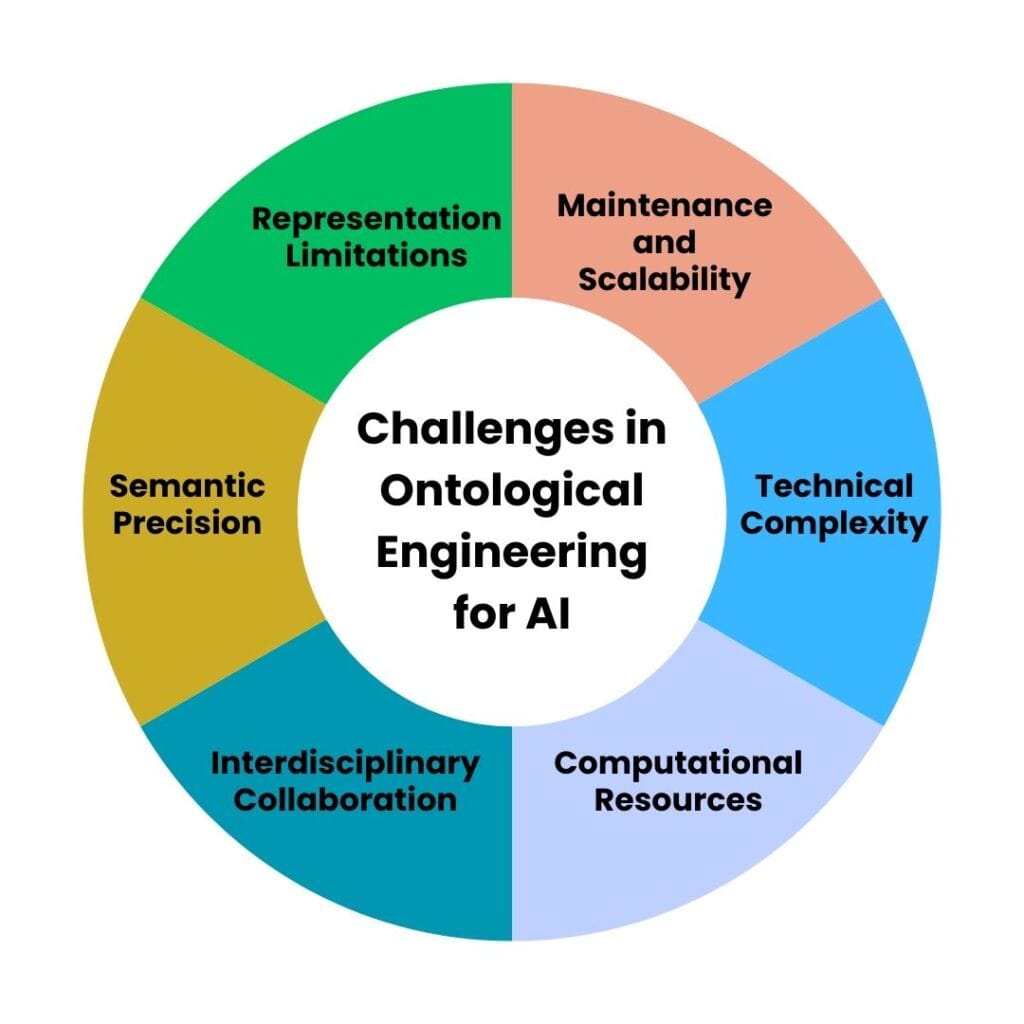
Developing ontologies for artificial intelligence presents several complex challenges that researchers and engineers must navigate carefully:
Technical Complexity
Creating accurate and comprehensive knowledge representations is inherently difficult. Ontologies require intricate mapping of relationships between concepts, demanding deep domain expertise and precise semantic modeling.
Maintenance and Scalability
As knowledge domains evolve rapidly, maintaining ontological frameworks becomes increasingly challenging. AI systems must continuously update and adapt their knowledge structures to remain relevant and accurate.
Interdisciplinary Collaboration
Effective ontological engineering requires collaboration across multiple disciplines, including:
- Computer science
- Linguistics
- Domain-specific expertise
- Cognitive science
Representation Limitations
Current ontological approaches struggle with:
- Capturing nuanced, context-dependent knowledge
- Handling ambiguity and uncertainty
- Representing complex, dynamic relationships
- Ensuring universal applicability across different domains
Computational Resources
Developing and implementing sophisticated ontologies demands significant computational power and advanced algorithmic techniques, which can be resource-intensive and expensive.
Semantic Precision
Achieving absolute precision in knowledge representation remains a significant challenge, as human knowledge is often subjective, contextual, and continuously evolving.
Future of Ontological Engineering in AI
Ontological engineering is poised for transformative advancements in artificial intelligence. Upcoming innovations will likely focus on creating more dynamic, adaptive knowledge representation systems that can learn and evolve in real time.
Key Future Developments
Anticipated progress includes:
- Advanced semantic modeling techniques
- More sophisticated machine learning integration
- Enhanced natural language understanding
- Improved cross-domain knowledge transfer
Potential Breakthroughs
Future ontological engineering may enable:
- More contextually aware AI systems
- Self-updating knowledge frameworks
- Enhanced predictive reasoning capabilities
- Deeper understanding of complex, nuanced information
Interdisciplinary Integration
Emerging approaches will likely combine insights from:
- Cognitive science
- Linguistics
- Data science
- Machine learning
Technological Enablers
Cutting-edge technologies will drive ontological engineering:
- Quantum computing
- Advanced neural networks
- Sophisticated machine learning algorithms
- Improved computational architectures
The future of ontological engineering promises more intelligent, adaptable, and context-aware artificial intelligence systems that can process and understand information with unprecedented depth and precision.
Conclusion
In conclusion, Ontological Engineering in AI represents a powerful approach to transforming how artificial intelligence understands and processes knowledge. By creating structured frameworks that enable machines to reason and interpret complex information, this innovative field continues to push the boundaries of intelligent systems, promising more sophisticated, adaptable, and context-aware AI technologies that can revolutionize multiple domains of human understanding and technological innovation.