Artificial Intelligence (AI) is becoming increasingly important in our daily lives. There are different types of AI, including generative AI and predictive AI.
Generative AI is capable of creating new data, such as text, images, or even music, based on the data it has been trained on. Predictive AI, on the other hand, analyzes existing data to make predictions or decisions.
Understanding the differences between generative AI vs predictive AI is crucial for effectively implementing AI solutions in various industries. Let’s dive deeper into what makes generative AI and predictive AI unique.
What is Generative AI?
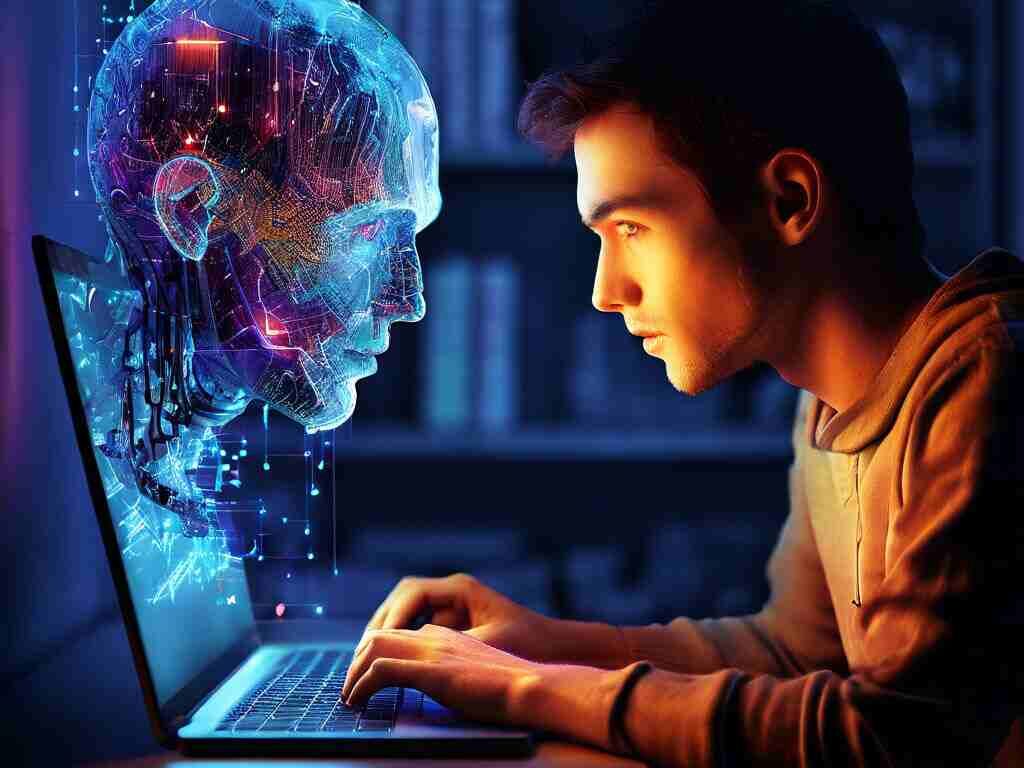
Generative AI is a type of artificial intelligence that can create new data like text, images, or audio from the data it was trained on. It uses machine learning to learn patterns and then generates unique content similar to the training data.
For example, a generative AI model can produce new pieces of writing or create realistic digital images based on text descriptions. Generative AI is considered “creative” because it produces novel outputs rather than just analyzing existing data.
What is Predictive AI?
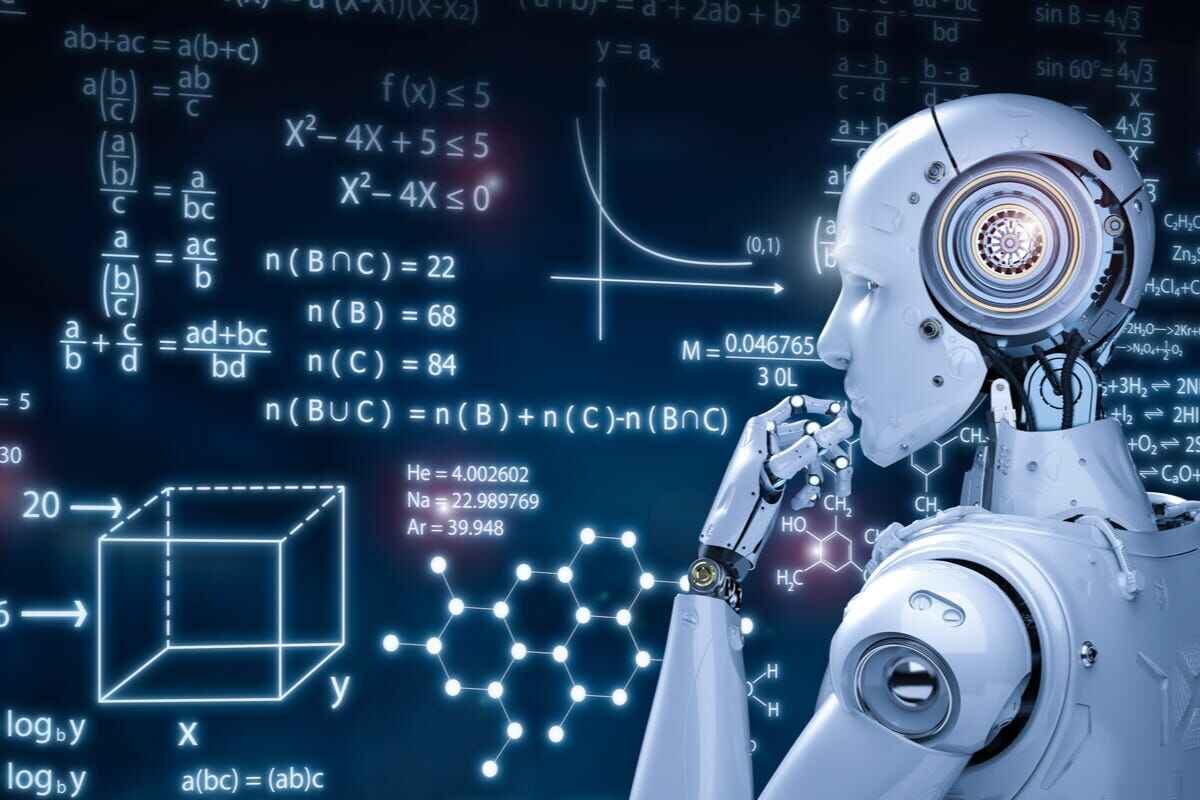
Predictive AI analyzes existing data to make predictions or decisions. It uses machine learning to find patterns and relationships in data to forecast future outcomes or suggest actions.
Common applications include recommendation systems that predict users’ preferences, fraud detection that identifies suspicious activities, and predictive maintenance that anticipates when equipment needs repairs.
Unlike generative AI which creates new data, predictive AI focuses on making informed predictions from available data.
Key Difference Between Generative AI vs Predictive AI
Here are the key differences between generative AI and predictive AI:
Purpose:
- Generative AI creates new data (text, images, audio, etc.)
- Predictive AI analyzes existing data to make predictions or decisions
Approach:
- Generative AI learns patterns from training data to generate new, unique outputs
- Predictive AI finds patterns and relationships in data to forecast future outcomes
Applications:
- Generative AI is used for creative tasks like writing, art, and content generation
- Predictive AI is used for analytical tasks like recommendations, fraud detection, and predictive maintenance
Nature:
- Generative AI is more open-ended and exploratory
- Predictive AI is more focused on specific tasks and decision-making
Output:
- Generative AI produces novel and unique data
- Predictive AI provides insights, predictions, or recommended actions
In summary, generative AI creates new content, while predictive AI analyzes data to make informed predictions or decisions. Their purposes, approaches, and applications differ, but both are valuable tools in the field of artificial intelligence.
Applications and Use Cases of Generative AI vs Predictive AI
Here are some key applications and use cases of generative AI and predictive AI, presented in a user-friendly manner:
Generative AI Applications:
- Content Creation: Generative AI can create unique articles, stories, scripts, and other written content.
- Digital Art and Design: It can generate realistic images, animations, and designs based on text descriptions or examples.
- Music and Audio Generation: Generative AI models can compose original music, sound effects, and audio clips.
- Gaming and Virtual Worlds: It can generate realistic environments, characters, and narratives for video games and virtual reality experiences.
Predictive AI Applications:
- Recommendation Systems: Predictive AI powers personalized recommendations for products, movies, shows, and content on e-commerce and streaming platforms.
- Fraud Detection: It analyzes transactions, user behavior, and other data to identify and prevent fraudulent activities in finance and cybersecurity.
- Predictive Maintenance: Predictive AI monitors machine data to predict when equipment or systems might need maintenance or repairs, reducing downtime.
- Forecasting and Analytics: It predicts future trends, demand, and outcomes based on historical data, aiding decision-making in various industries.
- Healthcare: Predictive AI can help diagnose diseases, predict patient outcomes, and personalize treatment plans based on patient data.
Both generative and predictive AI have numerous applications across various domains, from creative industries to data-driven decision-making. As the technology evolves, we can expect to see even more innovative and practical use cases emerge.
Future Aspects of Generative AI and Predictive AI
The fields of generative AI and predictive AI are rapidly evolving, and we can expect to see significant advancements in the coming years. Here are some potential future prospects:
Future Aspects of Generative AI:
- Improved quality and realism: Generative models will become more sophisticated, producing even more realistic and high-quality content that is indistinguishable from human-created output.
- Multimodal generation: Generative AI will be able to create content across multiple modalities simultaneously, such as generating text, images, and audio together.
- Personalized content creation: Generative AI will adapt to individual preferences and styles, enabling highly personalized content generation tailored to specific users or scenarios.
Future Aspects of Predictive AI:
- Increased accuracy and reliability: Predictive models will become more accurate and reliable as more data becomes available and algorithms continue to improve.
- Real-time predictions: Predictive AI will be able to make predictions in real-time, enabling instant decision-making and actionable insights.
- Explainable AI: There will be a focus on developing predictive AI systems that can explain their decision-making process, increasing transparency and trust.
- Integration with other technologies: Predictive AI will be integrated with other emerging technologies like the Internet of Things (IoT) and edge computing, enabling more efficient data processing and predictions.
Overall, both generative and predictive AI are expected to become more powerful, efficient, and widely adopted across various industries and applications. However, it is crucial to address ethical concerns, such as privacy, bias, and transparency, to ensure the responsible development and deployment of these technologies.
Conclusion
In conclusion, generative AI and predictive AI are two distinct types of artificial intelligence with different purposes and applications. Generative AI creates new data, while predictive AI analyzes existing data to make predictions or decisions. Understanding their differences is crucial for effectively utilizing these technologies in various domains. As AI continues to advance, we can expect to see more innovative and practical applications of both generative and predictive AI in the future.
4 thoughts on “Generative AI vs Predictive AI: Key Differences Explained”